What is AI Safety and How It is Achieved?
5 min read
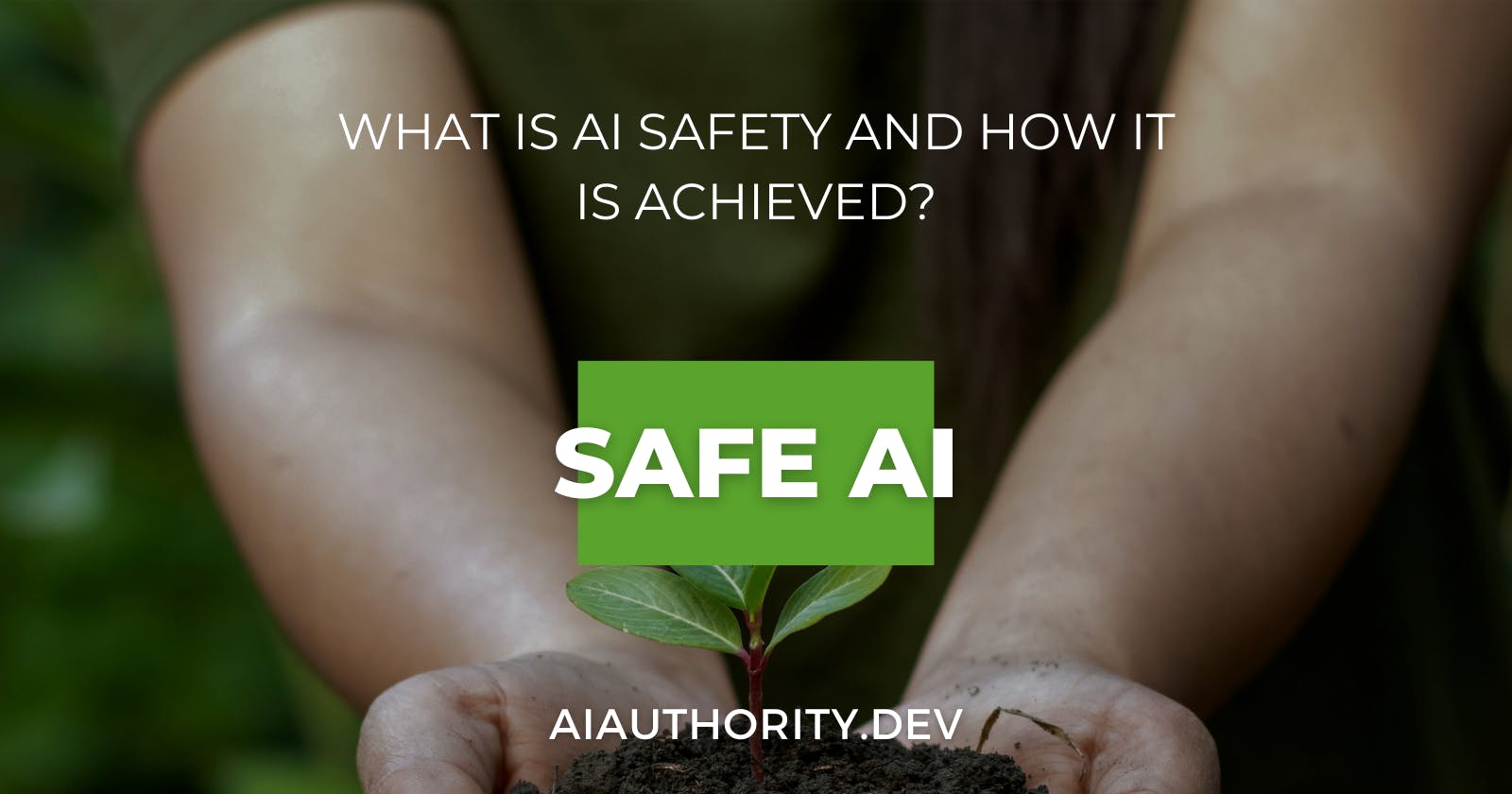
What is AI safety? Artificial intelligence safety definition
The term Artificial Intelligence Safety or AI Safety corresponds to a collection of guidelines and procedures designed to guarantee the responsible and secure development, implementation and use of artificial intelligence (AI) models and systems.
It includes a range of actions and ideas aimed at reducing possible hazards and unexpected effects related to AI technologies.
The field of AI Safety has gained increasing prominence in recent years as AI technologies have become more prevalent in society.
With the rapid advancement of AI capabilities, concerns have emerged regarding the potential for AI systems to exhibit harmful behaviors or unintended outcomes. These concerns encompass a range of issues, including bias in AI algorithms, security vulnerabilities, ethical dilemmas and the potential for AI systems to malfunction or make incorrect decisions.
To address these challenges, researchers, policymakers and industry stakeholders have emphasized the importance of integrating safety considerations into the design, development and deployment of AI systems. This involves adopting rigorous testing procedures, implementing safeguards to prevent bias and discrimination and establishing ethical guidelines for the responsible use of AI.
One of the key areas of focus in AI Safety is the identification and mitigation of risks associated with AI models.
These risks can include model poisoning, where malicious actors manipulate training data to compromise the integrity of AI models and bias, which can result in discriminatory outcomes. Additionally, concerns have been raised about the potential for AI systems to generate false or misleading information, a phenomenon known as "hallucination."
6 common AI risks that would need immediate attention in AI safety
The most immediate AI-related risks are present within the AI and prompt model. These can include:
- Model poisoning
Malicious actors may compromise the AI model learning process by injecting the training dataset with false and misleading data. Consequently, the model will learn and adapt these incorrect patterns, which will affect the generated outcomes.
- Bias
The AI model may generate outputs owing to the discriminatory data and assumptions that were part of the compromised dataset it was trained on. These biased outputs can lead to adverse consequences, especially in instances where the compromised AI model is being used for critical decision-making purposes.
- Hallucination
Hallucination refers to an output generated by an AI model that is either wholly false or corrupted. However, since the output is coherent and may follow a series of outputs that were not false, they may be harder to spot and identify.
- Prompt Injection
An input prompt is manipulated with the purpose of compromising an AI model’s outputs. If successful, a prompt injection will lead to false, biased and misleading responses from the AI model since the training dataset it is trained on will have been manipulated to trigger such a result.
- Prompt DoS
A Denial of Service (DoS) attack can be launched against an AI model to crash it. It is done by triggering an automated response within the model that has been trained on a manipulated dataset. The response can be triggered via the use of a compromised prompt intended to start a chain of events leading to eventual overload and crash of the model.
- Exfiltration Risks
Exfiltration risks refer to the ability of malicious actors to exploit certain words, phrases and terminologies to reverse engineer and leak training data. Information retrieved from such reverse engineering can then be further used to exploit potentially sensitive data. Such actors can deploy a combination of prompt injections and DoS attacks to this end.
Also Read: 5 AI communities to learn more about AI risks and AI safety
How to achieve AI safety to mitigate AI risks?
Proactive safety precautions are necessary due to the potential threats posed by AI systems as they become more sophisticated and self-governing.
The AI development community is striving to reduce risks and increase trust in this potent technology through stringent testing, supervision, transparency and control.
Algorithmic audits detect biases or unfair results in order to identify problems in testing environments early on.
Additionally, in order to mathematically validate properties like privacy and reliability, researchers create formal verification methods. These checks remain critical as AI models grow in size and complexity.
Once deployed, continuous monitoring and logging of AI choices enables for the detection of abnormalities or errors, which can then be corrected promptly.
"Constitutional" AI techniques seek to connect technological systems with ethical ideals such as safety and fairness by design.
Here are a few more solutions for mitigating the risks in AI and prompt models.
To identify and stop the introduction of erroneous or misleading data, enforce stringent data validation procedures and conduct routine audits of training datasets.
To reduce the likelihood that AI models may produce biased results, use representative and diverse datasets in conjunction with bias detection methods.
Create strong anomaly detection systems to spot and mark erroneous or tainted outputs produced by AI models.
To guard against manipulation and guarantee the integrity of AI model outputs, use encryption techniques and secure input prompts.
To prevent malicious parties from using AI training data for their own purposes, strengthen data security measures and put encryption methods in place.
Robust in-line data and AI controls, like anonymization of data before providing it to AI models, entitlement controls and LLM firewalls, can be used by organizations to enforce compliance with security, privacy and governance policies, securing sensitive data throughout its lifecycle.
Also read: Strengthening the security of LLM models
Wrapping up
AI safety is the umbrella term for a variety of practices and procedures an organization uses to guarantee AI systems function safely and as intended, reducing the possibility of harm or unexpected repercussions.
The pursuit of AI safety is a journey that will call for consistent commitment and receptiveness from a wide range of global players.
If you want to add any comment or input, please do it in the comments section below. We can surely come by and dig into it.
References:
Open AI’s approach to AI safety
AI safety vs control vs alignment